Merging a huge list of dataframes using dask delayed
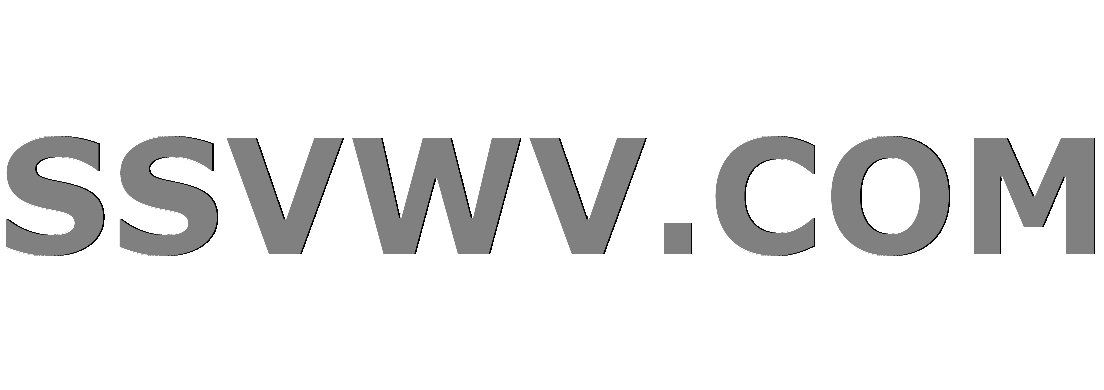
Multi tool use
up vote
0
down vote
favorite
I have a function which returns a dataframe to me. I am trying to use this function in parallel by using dask.
I append the delayed objects of the dataframes into a list. However, the run-time of my code is the same with and without dask.delayed.
I use the reduce function from functools along with pd.merge
to merge my dataframes.
Any suggestions on how to improve the run-time?
The visualized graph and code are as below.
from functools import reduce
d =
for lot in lots:
lot_data = data[data["LOTID"]==lot]
trmat = delayed(LOT)(lot, lot_data).transition_matrix(lot)
d.append(trmat)
df = delayed(reduce)(lambda x, y: x.merge(y, how='outer', on=['from', "to"]), d)
Visualized graph of the operations
dask dask-delayed
add a comment |
up vote
0
down vote
favorite
I have a function which returns a dataframe to me. I am trying to use this function in parallel by using dask.
I append the delayed objects of the dataframes into a list. However, the run-time of my code is the same with and without dask.delayed.
I use the reduce function from functools along with pd.merge
to merge my dataframes.
Any suggestions on how to improve the run-time?
The visualized graph and code are as below.
from functools import reduce
d =
for lot in lots:
lot_data = data[data["LOTID"]==lot]
trmat = delayed(LOT)(lot, lot_data).transition_matrix(lot)
d.append(trmat)
df = delayed(reduce)(lambda x, y: x.merge(y, how='outer', on=['from', "to"]), d)
Visualized graph of the operations
dask dask-delayed
add a comment |
up vote
0
down vote
favorite
up vote
0
down vote
favorite
I have a function which returns a dataframe to me. I am trying to use this function in parallel by using dask.
I append the delayed objects of the dataframes into a list. However, the run-time of my code is the same with and without dask.delayed.
I use the reduce function from functools along with pd.merge
to merge my dataframes.
Any suggestions on how to improve the run-time?
The visualized graph and code are as below.
from functools import reduce
d =
for lot in lots:
lot_data = data[data["LOTID"]==lot]
trmat = delayed(LOT)(lot, lot_data).transition_matrix(lot)
d.append(trmat)
df = delayed(reduce)(lambda x, y: x.merge(y, how='outer', on=['from', "to"]), d)
Visualized graph of the operations
dask dask-delayed
I have a function which returns a dataframe to me. I am trying to use this function in parallel by using dask.
I append the delayed objects of the dataframes into a list. However, the run-time of my code is the same with and without dask.delayed.
I use the reduce function from functools along with pd.merge
to merge my dataframes.
Any suggestions on how to improve the run-time?
The visualized graph and code are as below.
from functools import reduce
d =
for lot in lots:
lot_data = data[data["LOTID"]==lot]
trmat = delayed(LOT)(lot, lot_data).transition_matrix(lot)
d.append(trmat)
df = delayed(reduce)(lambda x, y: x.merge(y, how='outer', on=['from', "to"]), d)
Visualized graph of the operations
dask dask-delayed
dask dask-delayed
edited Nov 12 at 8:01
asked Nov 11 at 19:46


NIMA MANAFZADEH DIZBIN
11
11
add a comment |
add a comment |
1 Answer
1
active
oldest
votes
up vote
0
down vote
General rule: if your data comfortable fits into memory (including the base size times a small number for possible intermediates), then there is a good chance that Pandas is fast and efficient for your use case.
Specifically for your case, there is a good chance that the tasks you are trying to parallelise do not release python's internal lock, the GIL, in which case although you have independent threads, only one can run at a time. The solution would be to use the "distributed" scheduler instead, which can have any mix of multiple threads and processed; however using processes comes at a cost for moving data between client and processes, and you may find that the extra cost dominates any time saving. You would certainly want to ensure that you load the data within the workers rather than passing from the client.
Short story, you should do some experimentation, measure well, and read the data-frame and distributed scheduler documentation carefully.
add a comment |
Your Answer
StackExchange.ifUsing("editor", function ()
StackExchange.using("externalEditor", function ()
StackExchange.using("snippets", function ()
StackExchange.snippets.init();
);
);
, "code-snippets");
StackExchange.ready(function()
var channelOptions =
tags: "".split(" "),
id: "1"
;
initTagRenderer("".split(" "), "".split(" "), channelOptions);
StackExchange.using("externalEditor", function()
// Have to fire editor after snippets, if snippets enabled
if (StackExchange.settings.snippets.snippetsEnabled)
StackExchange.using("snippets", function()
createEditor();
);
else
createEditor();
);
function createEditor()
StackExchange.prepareEditor(
heartbeatType: 'answer',
convertImagesToLinks: true,
noModals: true,
showLowRepImageUploadWarning: true,
reputationToPostImages: 10,
bindNavPrevention: true,
postfix: "",
imageUploader:
brandingHtml: "Powered by u003ca class="icon-imgur-white" href="https://imgur.com/"u003eu003c/au003e",
contentPolicyHtml: "User contributions licensed under u003ca href="https://creativecommons.org/licenses/by-sa/3.0/"u003ecc by-sa 3.0 with attribution requiredu003c/au003e u003ca href="https://stackoverflow.com/legal/content-policy"u003e(content policy)u003c/au003e",
allowUrls: true
,
onDemand: true,
discardSelector: ".discard-answer"
,immediatelyShowMarkdownHelp:true
);
);
Sign up or log in
StackExchange.ready(function ()
StackExchange.helpers.onClickDraftSave('#login-link');
);
Sign up using Google
Sign up using Facebook
Sign up using Email and Password
Post as a guest
Required, but never shown
StackExchange.ready(
function ()
StackExchange.openid.initPostLogin('.new-post-login', 'https%3a%2f%2fstackoverflow.com%2fquestions%2f53252539%2fmerging-a-huge-list-of-dataframes-using-dask-delayed%23new-answer', 'question_page');
);
Post as a guest
Required, but never shown
1 Answer
1
active
oldest
votes
1 Answer
1
active
oldest
votes
active
oldest
votes
active
oldest
votes
up vote
0
down vote
General rule: if your data comfortable fits into memory (including the base size times a small number for possible intermediates), then there is a good chance that Pandas is fast and efficient for your use case.
Specifically for your case, there is a good chance that the tasks you are trying to parallelise do not release python's internal lock, the GIL, in which case although you have independent threads, only one can run at a time. The solution would be to use the "distributed" scheduler instead, which can have any mix of multiple threads and processed; however using processes comes at a cost for moving data between client and processes, and you may find that the extra cost dominates any time saving. You would certainly want to ensure that you load the data within the workers rather than passing from the client.
Short story, you should do some experimentation, measure well, and read the data-frame and distributed scheduler documentation carefully.
add a comment |
up vote
0
down vote
General rule: if your data comfortable fits into memory (including the base size times a small number for possible intermediates), then there is a good chance that Pandas is fast and efficient for your use case.
Specifically for your case, there is a good chance that the tasks you are trying to parallelise do not release python's internal lock, the GIL, in which case although you have independent threads, only one can run at a time. The solution would be to use the "distributed" scheduler instead, which can have any mix of multiple threads and processed; however using processes comes at a cost for moving data between client and processes, and you may find that the extra cost dominates any time saving. You would certainly want to ensure that you load the data within the workers rather than passing from the client.
Short story, you should do some experimentation, measure well, and read the data-frame and distributed scheduler documentation carefully.
add a comment |
up vote
0
down vote
up vote
0
down vote
General rule: if your data comfortable fits into memory (including the base size times a small number for possible intermediates), then there is a good chance that Pandas is fast and efficient for your use case.
Specifically for your case, there is a good chance that the tasks you are trying to parallelise do not release python's internal lock, the GIL, in which case although you have independent threads, only one can run at a time. The solution would be to use the "distributed" scheduler instead, which can have any mix of multiple threads and processed; however using processes comes at a cost for moving data between client and processes, and you may find that the extra cost dominates any time saving. You would certainly want to ensure that you load the data within the workers rather than passing from the client.
Short story, you should do some experimentation, measure well, and read the data-frame and distributed scheduler documentation carefully.
General rule: if your data comfortable fits into memory (including the base size times a small number for possible intermediates), then there is a good chance that Pandas is fast and efficient for your use case.
Specifically for your case, there is a good chance that the tasks you are trying to parallelise do not release python's internal lock, the GIL, in which case although you have independent threads, only one can run at a time. The solution would be to use the "distributed" scheduler instead, which can have any mix of multiple threads and processed; however using processes comes at a cost for moving data between client and processes, and you may find that the extra cost dominates any time saving. You would certainly want to ensure that you load the data within the workers rather than passing from the client.
Short story, you should do some experimentation, measure well, and read the data-frame and distributed scheduler documentation carefully.
answered Nov 18 at 15:49


mdurant
9,79111435
9,79111435
add a comment |
add a comment |
Thanks for contributing an answer to Stack Overflow!
- Please be sure to answer the question. Provide details and share your research!
But avoid …
- Asking for help, clarification, or responding to other answers.
- Making statements based on opinion; back them up with references or personal experience.
To learn more, see our tips on writing great answers.
Some of your past answers have not been well-received, and you're in danger of being blocked from answering.
Please pay close attention to the following guidance:
- Please be sure to answer the question. Provide details and share your research!
But avoid …
- Asking for help, clarification, or responding to other answers.
- Making statements based on opinion; back them up with references or personal experience.
To learn more, see our tips on writing great answers.
Sign up or log in
StackExchange.ready(function ()
StackExchange.helpers.onClickDraftSave('#login-link');
);
Sign up using Google
Sign up using Facebook
Sign up using Email and Password
Post as a guest
Required, but never shown
StackExchange.ready(
function ()
StackExchange.openid.initPostLogin('.new-post-login', 'https%3a%2f%2fstackoverflow.com%2fquestions%2f53252539%2fmerging-a-huge-list-of-dataframes-using-dask-delayed%23new-answer', 'question_page');
);
Post as a guest
Required, but never shown
Sign up or log in
StackExchange.ready(function ()
StackExchange.helpers.onClickDraftSave('#login-link');
);
Sign up using Google
Sign up using Facebook
Sign up using Email and Password
Post as a guest
Required, but never shown
Sign up or log in
StackExchange.ready(function ()
StackExchange.helpers.onClickDraftSave('#login-link');
);
Sign up using Google
Sign up using Facebook
Sign up using Email and Password
Post as a guest
Required, but never shown
Sign up or log in
StackExchange.ready(function ()
StackExchange.helpers.onClickDraftSave('#login-link');
);
Sign up using Google
Sign up using Facebook
Sign up using Email and Password
Sign up using Google
Sign up using Facebook
Sign up using Email and Password
Post as a guest
Required, but never shown
Required, but never shown
Required, but never shown
Required, but never shown
Required, but never shown
Required, but never shown
Required, but never shown
Required, but never shown
Required, but never shown
htINQ SelauBZus0h66,pu5W8U9Dzlsy8W4gphJVLlT9zmm,fUIXcCDDF,fvz b9Bteqz2aQDfX4GxjDW5,KM0wIWW24i n6a