High accuracy measures when using pretrained embedding layer in Python
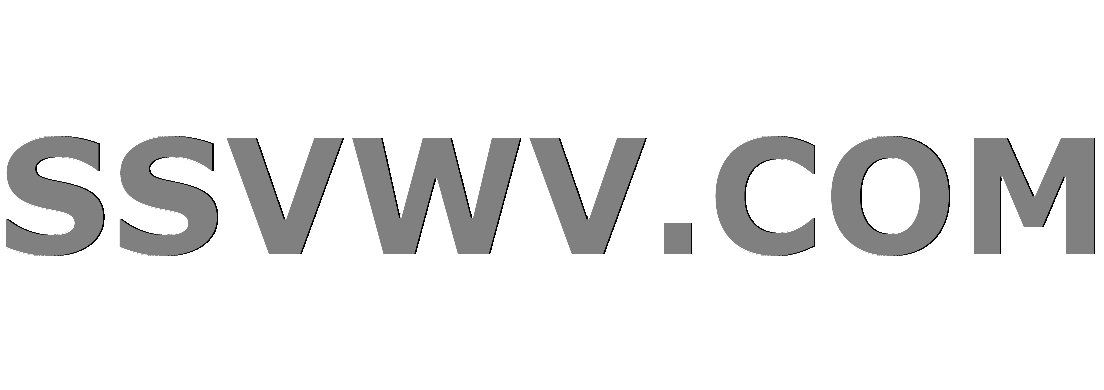
Multi tool use
I am trying to implement a pretrained embedding layer into my generative model using GloVe.
Into the model I feed sequences of 50 (X) items pulled from a text, and it is to predict the 51. word (y) in the text.
I reach an accuracy of 0.99 already when the model only has trained for 1/100 iterations. What can be the issue?
# create a weight matrix for words in training docs
embedding_matrix = zeros((vocab_size, 100))
for word, i in tokenizer.word_index.items():
embedding_vector = embeddings_index.get(word)
if embedding_vector is not None:
embedding_matrix[i] = embedding_vector
# define model
model = Sequential() #assigning the sequential function to a model
model.add(Embedding(vocab_size, 100, weights=[embedding_matrix], input_length=seq_length, trainable = False)) #defining embedding layer size
model.add(LSTM(100, return_sequences=True)) #adding layer of nodes
model.add(LSTM(100)) #adding layer of nodes
model.add(Dense(100, activation='relu')) #specifying the structure of the hidden layer, recu is an argument of a rectified linear unit.
model.add(Dense(vocab_size, activation='softmax')) #using the softmax function to creating probabilities
print(model.summary())
# compile the model
model.compile(optimizer='adam', loss='binary_crossentropy', metrics=['accuracy'])
# fit the model
model.fit(X, y, batch_size=128, epochs=100, verbose=1)
Link to github: https://github.com/KiriKoppelgaard/Generative_model
commit from Nov 14, 2018
python keras nlp
|
show 1 more comment
I am trying to implement a pretrained embedding layer into my generative model using GloVe.
Into the model I feed sequences of 50 (X) items pulled from a text, and it is to predict the 51. word (y) in the text.
I reach an accuracy of 0.99 already when the model only has trained for 1/100 iterations. What can be the issue?
# create a weight matrix for words in training docs
embedding_matrix = zeros((vocab_size, 100))
for word, i in tokenizer.word_index.items():
embedding_vector = embeddings_index.get(word)
if embedding_vector is not None:
embedding_matrix[i] = embedding_vector
# define model
model = Sequential() #assigning the sequential function to a model
model.add(Embedding(vocab_size, 100, weights=[embedding_matrix], input_length=seq_length, trainable = False)) #defining embedding layer size
model.add(LSTM(100, return_sequences=True)) #adding layer of nodes
model.add(LSTM(100)) #adding layer of nodes
model.add(Dense(100, activation='relu')) #specifying the structure of the hidden layer, recu is an argument of a rectified linear unit.
model.add(Dense(vocab_size, activation='softmax')) #using the softmax function to creating probabilities
print(model.summary())
# compile the model
model.compile(optimizer='adam', loss='binary_crossentropy', metrics=['accuracy'])
# fit the model
model.fit(X, y, batch_size=128, epochs=100, verbose=1)
Link to github: https://github.com/KiriKoppelgaard/Generative_model
commit from Nov 14, 2018
python keras nlp
What is the size of the corpus your are training on? Given the accuracy achieved you are very likely over-fitting the model due to a small dataset.
– sophros
Nov 15 '18 at 14:47
Is it the case that one sample may belong to multiple classes, i.e. be multiple words? I don't guess so.
– today
Nov 15 '18 at 14:49
I have around 500000 sequences the model is trained on, so I suspect it is not overfitting, but maybe I did not implement the embedding layer correctly.
– Kiri .Koppelgaard
Nov 15 '18 at 14:59
I am not sure I entirely get, what you are asking @today
– Kiri .Koppelgaard
Nov 15 '18 at 15:05
I am reffereing to the type of the model and the loss function you have used: if it is a single-label classification task (i.e. each sample has only one label and not mutiple label) it must becategorical_crossentropy
instead.
– today
Nov 15 '18 at 15:07
|
show 1 more comment
I am trying to implement a pretrained embedding layer into my generative model using GloVe.
Into the model I feed sequences of 50 (X) items pulled from a text, and it is to predict the 51. word (y) in the text.
I reach an accuracy of 0.99 already when the model only has trained for 1/100 iterations. What can be the issue?
# create a weight matrix for words in training docs
embedding_matrix = zeros((vocab_size, 100))
for word, i in tokenizer.word_index.items():
embedding_vector = embeddings_index.get(word)
if embedding_vector is not None:
embedding_matrix[i] = embedding_vector
# define model
model = Sequential() #assigning the sequential function to a model
model.add(Embedding(vocab_size, 100, weights=[embedding_matrix], input_length=seq_length, trainable = False)) #defining embedding layer size
model.add(LSTM(100, return_sequences=True)) #adding layer of nodes
model.add(LSTM(100)) #adding layer of nodes
model.add(Dense(100, activation='relu')) #specifying the structure of the hidden layer, recu is an argument of a rectified linear unit.
model.add(Dense(vocab_size, activation='softmax')) #using the softmax function to creating probabilities
print(model.summary())
# compile the model
model.compile(optimizer='adam', loss='binary_crossentropy', metrics=['accuracy'])
# fit the model
model.fit(X, y, batch_size=128, epochs=100, verbose=1)
Link to github: https://github.com/KiriKoppelgaard/Generative_model
commit from Nov 14, 2018
python keras nlp
I am trying to implement a pretrained embedding layer into my generative model using GloVe.
Into the model I feed sequences of 50 (X) items pulled from a text, and it is to predict the 51. word (y) in the text.
I reach an accuracy of 0.99 already when the model only has trained for 1/100 iterations. What can be the issue?
# create a weight matrix for words in training docs
embedding_matrix = zeros((vocab_size, 100))
for word, i in tokenizer.word_index.items():
embedding_vector = embeddings_index.get(word)
if embedding_vector is not None:
embedding_matrix[i] = embedding_vector
# define model
model = Sequential() #assigning the sequential function to a model
model.add(Embedding(vocab_size, 100, weights=[embedding_matrix], input_length=seq_length, trainable = False)) #defining embedding layer size
model.add(LSTM(100, return_sequences=True)) #adding layer of nodes
model.add(LSTM(100)) #adding layer of nodes
model.add(Dense(100, activation='relu')) #specifying the structure of the hidden layer, recu is an argument of a rectified linear unit.
model.add(Dense(vocab_size, activation='softmax')) #using the softmax function to creating probabilities
print(model.summary())
# compile the model
model.compile(optimizer='adam', loss='binary_crossentropy', metrics=['accuracy'])
# fit the model
model.fit(X, y, batch_size=128, epochs=100, verbose=1)
Link to github: https://github.com/KiriKoppelgaard/Generative_model
commit from Nov 14, 2018
python keras nlp
python keras nlp
edited Nov 15 '18 at 15:04
Kiri .Koppelgaard
asked Nov 15 '18 at 14:42


Kiri .KoppelgaardKiri .Koppelgaard
11
11
What is the size of the corpus your are training on? Given the accuracy achieved you are very likely over-fitting the model due to a small dataset.
– sophros
Nov 15 '18 at 14:47
Is it the case that one sample may belong to multiple classes, i.e. be multiple words? I don't guess so.
– today
Nov 15 '18 at 14:49
I have around 500000 sequences the model is trained on, so I suspect it is not overfitting, but maybe I did not implement the embedding layer correctly.
– Kiri .Koppelgaard
Nov 15 '18 at 14:59
I am not sure I entirely get, what you are asking @today
– Kiri .Koppelgaard
Nov 15 '18 at 15:05
I am reffereing to the type of the model and the loss function you have used: if it is a single-label classification task (i.e. each sample has only one label and not mutiple label) it must becategorical_crossentropy
instead.
– today
Nov 15 '18 at 15:07
|
show 1 more comment
What is the size of the corpus your are training on? Given the accuracy achieved you are very likely over-fitting the model due to a small dataset.
– sophros
Nov 15 '18 at 14:47
Is it the case that one sample may belong to multiple classes, i.e. be multiple words? I don't guess so.
– today
Nov 15 '18 at 14:49
I have around 500000 sequences the model is trained on, so I suspect it is not overfitting, but maybe I did not implement the embedding layer correctly.
– Kiri .Koppelgaard
Nov 15 '18 at 14:59
I am not sure I entirely get, what you are asking @today
– Kiri .Koppelgaard
Nov 15 '18 at 15:05
I am reffereing to the type of the model and the loss function you have used: if it is a single-label classification task (i.e. each sample has only one label and not mutiple label) it must becategorical_crossentropy
instead.
– today
Nov 15 '18 at 15:07
What is the size of the corpus your are training on? Given the accuracy achieved you are very likely over-fitting the model due to a small dataset.
– sophros
Nov 15 '18 at 14:47
What is the size of the corpus your are training on? Given the accuracy achieved you are very likely over-fitting the model due to a small dataset.
– sophros
Nov 15 '18 at 14:47
Is it the case that one sample may belong to multiple classes, i.e. be multiple words? I don't guess so.
– today
Nov 15 '18 at 14:49
Is it the case that one sample may belong to multiple classes, i.e. be multiple words? I don't guess so.
– today
Nov 15 '18 at 14:49
I have around 500000 sequences the model is trained on, so I suspect it is not overfitting, but maybe I did not implement the embedding layer correctly.
– Kiri .Koppelgaard
Nov 15 '18 at 14:59
I have around 500000 sequences the model is trained on, so I suspect it is not overfitting, but maybe I did not implement the embedding layer correctly.
– Kiri .Koppelgaard
Nov 15 '18 at 14:59
I am not sure I entirely get, what you are asking @today
– Kiri .Koppelgaard
Nov 15 '18 at 15:05
I am not sure I entirely get, what you are asking @today
– Kiri .Koppelgaard
Nov 15 '18 at 15:05
I am reffereing to the type of the model and the loss function you have used: if it is a single-label classification task (i.e. each sample has only one label and not mutiple label) it must be
categorical_crossentropy
instead.– today
Nov 15 '18 at 15:07
I am reffereing to the type of the model and the loss function you have used: if it is a single-label classification task (i.e. each sample has only one label and not mutiple label) it must be
categorical_crossentropy
instead.– today
Nov 15 '18 at 15:07
|
show 1 more comment
0
active
oldest
votes
Your Answer
StackExchange.ifUsing("editor", function ()
StackExchange.using("externalEditor", function ()
StackExchange.using("snippets", function ()
StackExchange.snippets.init();
);
);
, "code-snippets");
StackExchange.ready(function()
var channelOptions =
tags: "".split(" "),
id: "1"
;
initTagRenderer("".split(" "), "".split(" "), channelOptions);
StackExchange.using("externalEditor", function()
// Have to fire editor after snippets, if snippets enabled
if (StackExchange.settings.snippets.snippetsEnabled)
StackExchange.using("snippets", function()
createEditor();
);
else
createEditor();
);
function createEditor()
StackExchange.prepareEditor(
heartbeatType: 'answer',
autoActivateHeartbeat: false,
convertImagesToLinks: true,
noModals: true,
showLowRepImageUploadWarning: true,
reputationToPostImages: 10,
bindNavPrevention: true,
postfix: "",
imageUploader:
brandingHtml: "Powered by u003ca class="icon-imgur-white" href="https://imgur.com/"u003eu003c/au003e",
contentPolicyHtml: "User contributions licensed under u003ca href="https://creativecommons.org/licenses/by-sa/3.0/"u003ecc by-sa 3.0 with attribution requiredu003c/au003e u003ca href="https://stackoverflow.com/legal/content-policy"u003e(content policy)u003c/au003e",
allowUrls: true
,
onDemand: true,
discardSelector: ".discard-answer"
,immediatelyShowMarkdownHelp:true
);
);
Sign up or log in
StackExchange.ready(function ()
StackExchange.helpers.onClickDraftSave('#login-link');
);
Sign up using Google
Sign up using Facebook
Sign up using Email and Password
Post as a guest
Required, but never shown
StackExchange.ready(
function ()
StackExchange.openid.initPostLogin('.new-post-login', 'https%3a%2f%2fstackoverflow.com%2fquestions%2f53321913%2fhigh-accuracy-measures-when-using-pretrained-embedding-layer-in-python%23new-answer', 'question_page');
);
Post as a guest
Required, but never shown
0
active
oldest
votes
0
active
oldest
votes
active
oldest
votes
active
oldest
votes
Thanks for contributing an answer to Stack Overflow!
- Please be sure to answer the question. Provide details and share your research!
But avoid …
- Asking for help, clarification, or responding to other answers.
- Making statements based on opinion; back them up with references or personal experience.
To learn more, see our tips on writing great answers.
Sign up or log in
StackExchange.ready(function ()
StackExchange.helpers.onClickDraftSave('#login-link');
);
Sign up using Google
Sign up using Facebook
Sign up using Email and Password
Post as a guest
Required, but never shown
StackExchange.ready(
function ()
StackExchange.openid.initPostLogin('.new-post-login', 'https%3a%2f%2fstackoverflow.com%2fquestions%2f53321913%2fhigh-accuracy-measures-when-using-pretrained-embedding-layer-in-python%23new-answer', 'question_page');
);
Post as a guest
Required, but never shown
Sign up or log in
StackExchange.ready(function ()
StackExchange.helpers.onClickDraftSave('#login-link');
);
Sign up using Google
Sign up using Facebook
Sign up using Email and Password
Post as a guest
Required, but never shown
Sign up or log in
StackExchange.ready(function ()
StackExchange.helpers.onClickDraftSave('#login-link');
);
Sign up using Google
Sign up using Facebook
Sign up using Email and Password
Post as a guest
Required, but never shown
Sign up or log in
StackExchange.ready(function ()
StackExchange.helpers.onClickDraftSave('#login-link');
);
Sign up using Google
Sign up using Facebook
Sign up using Email and Password
Sign up using Google
Sign up using Facebook
Sign up using Email and Password
Post as a guest
Required, but never shown
Required, but never shown
Required, but never shown
Required, but never shown
Required, but never shown
Required, but never shown
Required, but never shown
Required, but never shown
Required, but never shown
CUgjfnc
What is the size of the corpus your are training on? Given the accuracy achieved you are very likely over-fitting the model due to a small dataset.
– sophros
Nov 15 '18 at 14:47
Is it the case that one sample may belong to multiple classes, i.e. be multiple words? I don't guess so.
– today
Nov 15 '18 at 14:49
I have around 500000 sequences the model is trained on, so I suspect it is not overfitting, but maybe I did not implement the embedding layer correctly.
– Kiri .Koppelgaard
Nov 15 '18 at 14:59
I am not sure I entirely get, what you are asking @today
– Kiri .Koppelgaard
Nov 15 '18 at 15:05
I am reffereing to the type of the model and the loss function you have used: if it is a single-label classification task (i.e. each sample has only one label and not mutiple label) it must be
categorical_crossentropy
instead.– today
Nov 15 '18 at 15:07