I'm getting a Memory Error while processing my dataset in python ? What could be the reason?
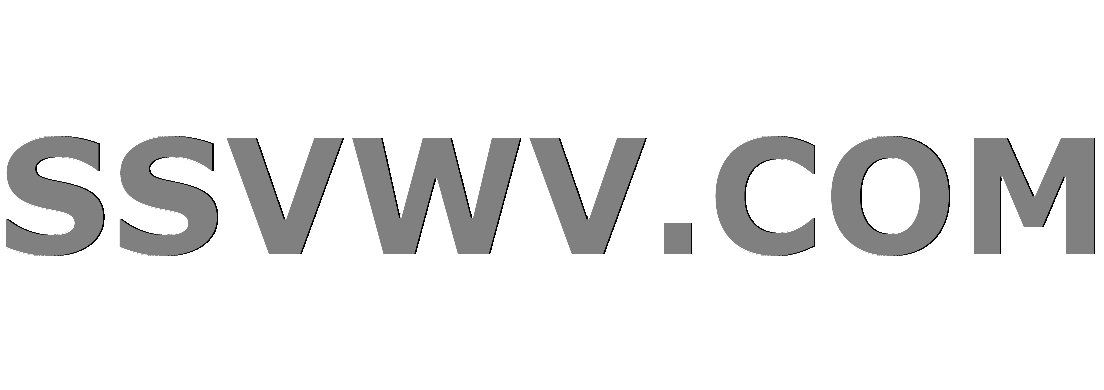
Multi tool use
up vote
2
down vote
favorite
I'm trying a deep learning code for processing my dataset which consists 1,12,120 images. What my code does is the following:
import numpy as np
import matplotlib.pyplot as plt
import pandas as pd
import imageio
from os import listdir
import skimage.transform
import pickle
import sys, os
from sklearn.preprocessing import MultiLabelBinarizer
def get_labels(pic_id):
labels = meta_data.loc[meta_data["Image Index"]==pic_id,"Finding Labels"]
return labels.tolist()[0].split("|")
#Loading Data
meta_data = pd.read_csv(data_entry_path)
bbox_list = pd.read_csv(bbox_list_path)
with open(train_txt_path, "r") as f:
train_list = [ i.strip() for i in f.readlines()]
with open(valid_txt_path, "r") as f:
valid_list = [ i.strip() for i in f.readlines()]
label_eight = list(np.unique(bbox_list["Finding Label"])) + ["No Finding"]
# transform training images
print("training example:",len(train_list))
print("take care of your RAM here !!!")
train_X =
for i in range(len(train_list)):
image_path = os.path.join(image_folder_path,train_list[i])
img = imageio.imread(image_path)
if img.shape != (1024,1024): # there some image with shape (1024,1024,4) in training set
img = img[:,:,0]
img_resized = skimage.transform.resize(img,(256,256)) # or use img[::4] here
train_X.append((np.array(img_resized)/255).reshape(256,256,1))
if i % 3000==0:
print(i)
train_X = np.array(train_X)
np.save(os.path.join(data_path,"train_X_small.npy"), train_X)
# transform validation images
print("validation example:",len(valid_list))
valid_X =
for i in range(len(valid_list)):
image_path = os.path.join(image_folder_path,valid_list[i])
img = imageio.imread(image_path)
if img.shape != (1024,1024):
img = img[:,:,0]
img_resized = skimage.transform.resize(img,(256,256))
# if img.shape != (1024,1024):
# train_X.append(img[:,:,0])
# else:
valid_X.append((np.array(img_resized)/255).reshape(256,256,1))
if i % 3000==0:
print(i)
valid_X = np.array(valid_X)
np.save(os.path.join(data_path,"valid_X_small.npy"), valid_X)
# process label
print("label preprocessing")
train_y =
for train_id in train_list:
train_y.append(get_labels(train_id))
valid_y =
for valid_id in valid_list:
valid_y.append(get_labels(valid_id))
encoder = MultiLabelBinarizer()
encoder.fit(train_y+valid_y)
train_y_onehot = encoder.transform(train_y)
valid_y_onehot = encoder.transform(valid_y)
train_y_onehot = np.delete(train_y_onehot, [2,3,5,6,7,10,12],1) # delete out 8 and "No Finding" column
valid_y_onehot = np.delete(valid_y_onehot, [2,3,5,6,7,10,12],1) # delete out 8 and "No Finding" column
with open(data_path + "/train_y_onehot.pkl","wb") as f:
pickle.dump(train_y_onehot, f)
with open(data_path + "/valid_y_onehot.pkl","wb") as f:
pickle.dump(valid_y_onehot, f)
with open(data_path + "/label_encoder.pkl","wb") as f:
pickle.dump(encoder, f)
So this is my code My system configration:Intel i7-7700HQ,16GB Ram,256GB ssd,GTX 1050 4GB
Is there a way to split my dataset so and write to the same file again? I'm also posting the error which i got as a screenshot Error From Powershell after executing the code for 30mins
I'm also using python3 in my system 64bit version
Does spliting the 1,12,120 images and taking them as batches will it work here? If yes how?
python python-3.x numpy deep-learning sklearn-pandas
|
show 5 more comments
up vote
2
down vote
favorite
I'm trying a deep learning code for processing my dataset which consists 1,12,120 images. What my code does is the following:
import numpy as np
import matplotlib.pyplot as plt
import pandas as pd
import imageio
from os import listdir
import skimage.transform
import pickle
import sys, os
from sklearn.preprocessing import MultiLabelBinarizer
def get_labels(pic_id):
labels = meta_data.loc[meta_data["Image Index"]==pic_id,"Finding Labels"]
return labels.tolist()[0].split("|")
#Loading Data
meta_data = pd.read_csv(data_entry_path)
bbox_list = pd.read_csv(bbox_list_path)
with open(train_txt_path, "r") as f:
train_list = [ i.strip() for i in f.readlines()]
with open(valid_txt_path, "r") as f:
valid_list = [ i.strip() for i in f.readlines()]
label_eight = list(np.unique(bbox_list["Finding Label"])) + ["No Finding"]
# transform training images
print("training example:",len(train_list))
print("take care of your RAM here !!!")
train_X =
for i in range(len(train_list)):
image_path = os.path.join(image_folder_path,train_list[i])
img = imageio.imread(image_path)
if img.shape != (1024,1024): # there some image with shape (1024,1024,4) in training set
img = img[:,:,0]
img_resized = skimage.transform.resize(img,(256,256)) # or use img[::4] here
train_X.append((np.array(img_resized)/255).reshape(256,256,1))
if i % 3000==0:
print(i)
train_X = np.array(train_X)
np.save(os.path.join(data_path,"train_X_small.npy"), train_X)
# transform validation images
print("validation example:",len(valid_list))
valid_X =
for i in range(len(valid_list)):
image_path = os.path.join(image_folder_path,valid_list[i])
img = imageio.imread(image_path)
if img.shape != (1024,1024):
img = img[:,:,0]
img_resized = skimage.transform.resize(img,(256,256))
# if img.shape != (1024,1024):
# train_X.append(img[:,:,0])
# else:
valid_X.append((np.array(img_resized)/255).reshape(256,256,1))
if i % 3000==0:
print(i)
valid_X = np.array(valid_X)
np.save(os.path.join(data_path,"valid_X_small.npy"), valid_X)
# process label
print("label preprocessing")
train_y =
for train_id in train_list:
train_y.append(get_labels(train_id))
valid_y =
for valid_id in valid_list:
valid_y.append(get_labels(valid_id))
encoder = MultiLabelBinarizer()
encoder.fit(train_y+valid_y)
train_y_onehot = encoder.transform(train_y)
valid_y_onehot = encoder.transform(valid_y)
train_y_onehot = np.delete(train_y_onehot, [2,3,5,6,7,10,12],1) # delete out 8 and "No Finding" column
valid_y_onehot = np.delete(valid_y_onehot, [2,3,5,6,7,10,12],1) # delete out 8 and "No Finding" column
with open(data_path + "/train_y_onehot.pkl","wb") as f:
pickle.dump(train_y_onehot, f)
with open(data_path + "/valid_y_onehot.pkl","wb") as f:
pickle.dump(valid_y_onehot, f)
with open(data_path + "/label_encoder.pkl","wb") as f:
pickle.dump(encoder, f)
So this is my code My system configration:Intel i7-7700HQ,16GB Ram,256GB ssd,GTX 1050 4GB
Is there a way to split my dataset so and write to the same file again? I'm also posting the error which i got as a screenshot Error From Powershell after executing the code for 30mins
I'm also using python3 in my system 64bit version
Does spliting the 1,12,120 images and taking them as batches will it work here? If yes how?
python python-3.x numpy deep-learning sklearn-pandas
1
A back of the envelope calculation says 78,000 * 256 * 256 * 3 = 15.5gb, so it seems that you're running out of memory right on schedule. There are options for dealing with this but they depend on what your overall goal is.
– CJ59
Nov 10 at 13:31
Is there anyway to overcome this ?
– Amrudesh Balakrishnan
Nov 10 at 13:41
Fix your formatting, and post a smaller proof of concept. stackoverflow.com/help/mcve
– Jim Stewart
Nov 10 at 13:44
This is the best I can do sir this is the same way how I wrote the code
– Amrudesh Balakrishnan
Nov 10 at 13:48
The trivial answer is to downsample your images more or to use fewer images. You could preprocess all your data, save it to a new file and memory map that file (but it would almost certainly work terribly). The real answer is that this is approaching an HPC scale problem.
– CJ59
Nov 10 at 13:50
|
show 5 more comments
up vote
2
down vote
favorite
up vote
2
down vote
favorite
I'm trying a deep learning code for processing my dataset which consists 1,12,120 images. What my code does is the following:
import numpy as np
import matplotlib.pyplot as plt
import pandas as pd
import imageio
from os import listdir
import skimage.transform
import pickle
import sys, os
from sklearn.preprocessing import MultiLabelBinarizer
def get_labels(pic_id):
labels = meta_data.loc[meta_data["Image Index"]==pic_id,"Finding Labels"]
return labels.tolist()[0].split("|")
#Loading Data
meta_data = pd.read_csv(data_entry_path)
bbox_list = pd.read_csv(bbox_list_path)
with open(train_txt_path, "r") as f:
train_list = [ i.strip() for i in f.readlines()]
with open(valid_txt_path, "r") as f:
valid_list = [ i.strip() for i in f.readlines()]
label_eight = list(np.unique(bbox_list["Finding Label"])) + ["No Finding"]
# transform training images
print("training example:",len(train_list))
print("take care of your RAM here !!!")
train_X =
for i in range(len(train_list)):
image_path = os.path.join(image_folder_path,train_list[i])
img = imageio.imread(image_path)
if img.shape != (1024,1024): # there some image with shape (1024,1024,4) in training set
img = img[:,:,0]
img_resized = skimage.transform.resize(img,(256,256)) # or use img[::4] here
train_X.append((np.array(img_resized)/255).reshape(256,256,1))
if i % 3000==0:
print(i)
train_X = np.array(train_X)
np.save(os.path.join(data_path,"train_X_small.npy"), train_X)
# transform validation images
print("validation example:",len(valid_list))
valid_X =
for i in range(len(valid_list)):
image_path = os.path.join(image_folder_path,valid_list[i])
img = imageio.imread(image_path)
if img.shape != (1024,1024):
img = img[:,:,0]
img_resized = skimage.transform.resize(img,(256,256))
# if img.shape != (1024,1024):
# train_X.append(img[:,:,0])
# else:
valid_X.append((np.array(img_resized)/255).reshape(256,256,1))
if i % 3000==0:
print(i)
valid_X = np.array(valid_X)
np.save(os.path.join(data_path,"valid_X_small.npy"), valid_X)
# process label
print("label preprocessing")
train_y =
for train_id in train_list:
train_y.append(get_labels(train_id))
valid_y =
for valid_id in valid_list:
valid_y.append(get_labels(valid_id))
encoder = MultiLabelBinarizer()
encoder.fit(train_y+valid_y)
train_y_onehot = encoder.transform(train_y)
valid_y_onehot = encoder.transform(valid_y)
train_y_onehot = np.delete(train_y_onehot, [2,3,5,6,7,10,12],1) # delete out 8 and "No Finding" column
valid_y_onehot = np.delete(valid_y_onehot, [2,3,5,6,7,10,12],1) # delete out 8 and "No Finding" column
with open(data_path + "/train_y_onehot.pkl","wb") as f:
pickle.dump(train_y_onehot, f)
with open(data_path + "/valid_y_onehot.pkl","wb") as f:
pickle.dump(valid_y_onehot, f)
with open(data_path + "/label_encoder.pkl","wb") as f:
pickle.dump(encoder, f)
So this is my code My system configration:Intel i7-7700HQ,16GB Ram,256GB ssd,GTX 1050 4GB
Is there a way to split my dataset so and write to the same file again? I'm also posting the error which i got as a screenshot Error From Powershell after executing the code for 30mins
I'm also using python3 in my system 64bit version
Does spliting the 1,12,120 images and taking them as batches will it work here? If yes how?
python python-3.x numpy deep-learning sklearn-pandas
I'm trying a deep learning code for processing my dataset which consists 1,12,120 images. What my code does is the following:
import numpy as np
import matplotlib.pyplot as plt
import pandas as pd
import imageio
from os import listdir
import skimage.transform
import pickle
import sys, os
from sklearn.preprocessing import MultiLabelBinarizer
def get_labels(pic_id):
labels = meta_data.loc[meta_data["Image Index"]==pic_id,"Finding Labels"]
return labels.tolist()[0].split("|")
#Loading Data
meta_data = pd.read_csv(data_entry_path)
bbox_list = pd.read_csv(bbox_list_path)
with open(train_txt_path, "r") as f:
train_list = [ i.strip() for i in f.readlines()]
with open(valid_txt_path, "r") as f:
valid_list = [ i.strip() for i in f.readlines()]
label_eight = list(np.unique(bbox_list["Finding Label"])) + ["No Finding"]
# transform training images
print("training example:",len(train_list))
print("take care of your RAM here !!!")
train_X =
for i in range(len(train_list)):
image_path = os.path.join(image_folder_path,train_list[i])
img = imageio.imread(image_path)
if img.shape != (1024,1024): # there some image with shape (1024,1024,4) in training set
img = img[:,:,0]
img_resized = skimage.transform.resize(img,(256,256)) # or use img[::4] here
train_X.append((np.array(img_resized)/255).reshape(256,256,1))
if i % 3000==0:
print(i)
train_X = np.array(train_X)
np.save(os.path.join(data_path,"train_X_small.npy"), train_X)
# transform validation images
print("validation example:",len(valid_list))
valid_X =
for i in range(len(valid_list)):
image_path = os.path.join(image_folder_path,valid_list[i])
img = imageio.imread(image_path)
if img.shape != (1024,1024):
img = img[:,:,0]
img_resized = skimage.transform.resize(img,(256,256))
# if img.shape != (1024,1024):
# train_X.append(img[:,:,0])
# else:
valid_X.append((np.array(img_resized)/255).reshape(256,256,1))
if i % 3000==0:
print(i)
valid_X = np.array(valid_X)
np.save(os.path.join(data_path,"valid_X_small.npy"), valid_X)
# process label
print("label preprocessing")
train_y =
for train_id in train_list:
train_y.append(get_labels(train_id))
valid_y =
for valid_id in valid_list:
valid_y.append(get_labels(valid_id))
encoder = MultiLabelBinarizer()
encoder.fit(train_y+valid_y)
train_y_onehot = encoder.transform(train_y)
valid_y_onehot = encoder.transform(valid_y)
train_y_onehot = np.delete(train_y_onehot, [2,3,5,6,7,10,12],1) # delete out 8 and "No Finding" column
valid_y_onehot = np.delete(valid_y_onehot, [2,3,5,6,7,10,12],1) # delete out 8 and "No Finding" column
with open(data_path + "/train_y_onehot.pkl","wb") as f:
pickle.dump(train_y_onehot, f)
with open(data_path + "/valid_y_onehot.pkl","wb") as f:
pickle.dump(valid_y_onehot, f)
with open(data_path + "/label_encoder.pkl","wb") as f:
pickle.dump(encoder, f)
So this is my code My system configration:Intel i7-7700HQ,16GB Ram,256GB ssd,GTX 1050 4GB
Is there a way to split my dataset so and write to the same file again? I'm also posting the error which i got as a screenshot Error From Powershell after executing the code for 30mins
I'm also using python3 in my system 64bit version
Does spliting the 1,12,120 images and taking them as batches will it work here? If yes how?
python python-3.x numpy deep-learning sklearn-pandas
python python-3.x numpy deep-learning sklearn-pandas
edited Nov 10 at 19:40
asked Nov 10 at 13:18


Amrudesh Balakrishnan
165
165
1
A back of the envelope calculation says 78,000 * 256 * 256 * 3 = 15.5gb, so it seems that you're running out of memory right on schedule. There are options for dealing with this but they depend on what your overall goal is.
– CJ59
Nov 10 at 13:31
Is there anyway to overcome this ?
– Amrudesh Balakrishnan
Nov 10 at 13:41
Fix your formatting, and post a smaller proof of concept. stackoverflow.com/help/mcve
– Jim Stewart
Nov 10 at 13:44
This is the best I can do sir this is the same way how I wrote the code
– Amrudesh Balakrishnan
Nov 10 at 13:48
The trivial answer is to downsample your images more or to use fewer images. You could preprocess all your data, save it to a new file and memory map that file (but it would almost certainly work terribly). The real answer is that this is approaching an HPC scale problem.
– CJ59
Nov 10 at 13:50
|
show 5 more comments
1
A back of the envelope calculation says 78,000 * 256 * 256 * 3 = 15.5gb, so it seems that you're running out of memory right on schedule. There are options for dealing with this but they depend on what your overall goal is.
– CJ59
Nov 10 at 13:31
Is there anyway to overcome this ?
– Amrudesh Balakrishnan
Nov 10 at 13:41
Fix your formatting, and post a smaller proof of concept. stackoverflow.com/help/mcve
– Jim Stewart
Nov 10 at 13:44
This is the best I can do sir this is the same way how I wrote the code
– Amrudesh Balakrishnan
Nov 10 at 13:48
The trivial answer is to downsample your images more or to use fewer images. You could preprocess all your data, save it to a new file and memory map that file (but it would almost certainly work terribly). The real answer is that this is approaching an HPC scale problem.
– CJ59
Nov 10 at 13:50
1
1
A back of the envelope calculation says 78,000 * 256 * 256 * 3 = 15.5gb, so it seems that you're running out of memory right on schedule. There are options for dealing with this but they depend on what your overall goal is.
– CJ59
Nov 10 at 13:31
A back of the envelope calculation says 78,000 * 256 * 256 * 3 = 15.5gb, so it seems that you're running out of memory right on schedule. There are options for dealing with this but they depend on what your overall goal is.
– CJ59
Nov 10 at 13:31
Is there anyway to overcome this ?
– Amrudesh Balakrishnan
Nov 10 at 13:41
Is there anyway to overcome this ?
– Amrudesh Balakrishnan
Nov 10 at 13:41
Fix your formatting, and post a smaller proof of concept. stackoverflow.com/help/mcve
– Jim Stewart
Nov 10 at 13:44
Fix your formatting, and post a smaller proof of concept. stackoverflow.com/help/mcve
– Jim Stewart
Nov 10 at 13:44
This is the best I can do sir this is the same way how I wrote the code
– Amrudesh Balakrishnan
Nov 10 at 13:48
This is the best I can do sir this is the same way how I wrote the code
– Amrudesh Balakrishnan
Nov 10 at 13:48
The trivial answer is to downsample your images more or to use fewer images. You could preprocess all your data, save it to a new file and memory map that file (but it would almost certainly work terribly). The real answer is that this is approaching an HPC scale problem.
– CJ59
Nov 10 at 13:50
The trivial answer is to downsample your images more or to use fewer images. You could preprocess all your data, save it to a new file and memory map that file (but it would almost certainly work terribly). The real answer is that this is approaching an HPC scale problem.
– CJ59
Nov 10 at 13:50
|
show 5 more comments
active
oldest
votes
active
oldest
votes
active
oldest
votes
active
oldest
votes
active
oldest
votes
Sign up or log in
StackExchange.ready(function ()
StackExchange.helpers.onClickDraftSave('#login-link');
);
Sign up using Google
Sign up using Facebook
Sign up using Email and Password
Post as a guest
Required, but never shown
StackExchange.ready(
function ()
StackExchange.openid.initPostLogin('.new-post-login', 'https%3a%2f%2fstackoverflow.com%2fquestions%2f53239342%2fim-getting-a-memory-error-while-processing-my-dataset-in-python-what-could-be%23new-answer', 'question_page');
);
Post as a guest
Required, but never shown
Sign up or log in
StackExchange.ready(function ()
StackExchange.helpers.onClickDraftSave('#login-link');
);
Sign up using Google
Sign up using Facebook
Sign up using Email and Password
Post as a guest
Required, but never shown
Sign up or log in
StackExchange.ready(function ()
StackExchange.helpers.onClickDraftSave('#login-link');
);
Sign up using Google
Sign up using Facebook
Sign up using Email and Password
Post as a guest
Required, but never shown
Sign up or log in
StackExchange.ready(function ()
StackExchange.helpers.onClickDraftSave('#login-link');
);
Sign up using Google
Sign up using Facebook
Sign up using Email and Password
Sign up using Google
Sign up using Facebook
Sign up using Email and Password
Post as a guest
Required, but never shown
Required, but never shown
Required, but never shown
Required, but never shown
Required, but never shown
Required, but never shown
Required, but never shown
Required, but never shown
Required, but never shown
Dbr,5jMyiwx2,B,DhuD 1elJ4VgOH,NyAPC P,NEZXE zN,AOM7y8CgFtA14FOocIqMphTav2g1e QMgm5ve5biAuc,0PKhx nt7tH lvh
1
A back of the envelope calculation says 78,000 * 256 * 256 * 3 = 15.5gb, so it seems that you're running out of memory right on schedule. There are options for dealing with this but they depend on what your overall goal is.
– CJ59
Nov 10 at 13:31
Is there anyway to overcome this ?
– Amrudesh Balakrishnan
Nov 10 at 13:41
Fix your formatting, and post a smaller proof of concept. stackoverflow.com/help/mcve
– Jim Stewart
Nov 10 at 13:44
This is the best I can do sir this is the same way how I wrote the code
– Amrudesh Balakrishnan
Nov 10 at 13:48
The trivial answer is to downsample your images more or to use fewer images. You could preprocess all your data, save it to a new file and memory map that file (but it would almost certainly work terribly). The real answer is that this is approaching an HPC scale problem.
– CJ59
Nov 10 at 13:50